09 Nov 2017
Do you have any behaviours that may impede safe driving such as driving when tired, making phone calls, watching videos, or even throwing things out of the window?
The Traffic Management Bureau of The Public Security Ministry of China reported that there were 304 million motor vehicles in China as of June 2017. Under these circumstances, detecting dangerous driving and promoting safety on public transportation is critical.
Shiyang Yan (pictured above), a PhD student from the Department of Computer Science and Software Engineering at Xi’an Jiaotong-Liverpool University, has carried out research under the supervision of Dr Bailing Zhang on deep learning, with a focus on the automatic recognition of unsafe driving behaviour and other surveillance tasks.
Deep learning is a new type of machine learning model that borrows some ideas from neural networks in the human brain. Aiming to understand and extract meaning from data, the past few years have witnessed a rapid development of deep learning models and successful applications to a large variety of problems ranging from image classification, to natural language processing and speech recognition. In certain areas such as face recognition, deep learning even outperforms humans.
By exploring the potential of different kinds of deep learning models, Shiyang’s study focused on action recognition within two different scenarios: static images and video streaming. Action recognition based on static images is usually regarded as an important part of image understanding, as Shiyang explained:
“Deep learning can make machines learn more expressive features during an on-going task,” said Shiyang. “Under most circumstances, we tend to choose recognition based on static images because this reduces calculation times and boosts efficiency of data processing,” said Shiyang.
A corresponding software system has already been developed that can also be used in some other operations such as animation, automatic video analysis, and surveillance.
“In real-life situations, we can combine this software with a monitoring system installed in a bus or truck,” Shiyang explained. “It uses algorithms to analyse the surveillance video footage in real-time. The dangerous behaviours of drivers can then be detected, for example, if they make a phone call, and a warning alarm will alert the driver that their driving behaviour is unsafe.”
“The technique can also be applied to other areas such as surveillance of drivers’ behaviour by traffic police,” he added.
Dr Bailing Zhang explained: “The system can recognise dangerous driving behaviours, such as making phone calls or driving while smoking or excessively tired, by analysing surveillance videos. This will efficiently assist local traffic management bureaus to detect dangerous driving and improve public transportation safety.”
“We are currently communicating with industrial partners in this field and hope to put this action-recognition software into practical use as soon as possible,” Dr Zhang added.
In addition to behaviour recognition, Shiyang also conducts research about detecting abnormal events for surveillance. He explained that around the world surveillance cameras film a lot of footage every day, and that automatic analysis techniques can provide assistance to the relevant departments to deal with these abnormal events.
Most of the videos recorded only contain ‘normal’ events and abnormal events are usually rare. Shiyang Yan’s research focuses on how to use a large amount of normal video data to learn a ‘normal pattern’, so that when abnormal things happen the system will sound an alarm that alerts relevant departments to handle the issues in a timely manner.
“It’s not an easy task to define or distinguish these ‘abnormal events’ from normal events so that a computer system can recognise them,” said Shiyang. "A good solution to our current problems is what’s called a ‘deep generative model’.
“This model can learn a probability distribution of a ‘normal’ mode and abnormal events that don’t match the probability distribution. Once something unusual happens, the software will sound an alarm," he explained.
In addition to action recognition and event detection Dr Zhang also leads students to extend research on deep learning into other areas, including identifying people and vehicles in surveillance videos, scene recognition, face recognition, and natural language processing such as automatic translation.
by Guojuan Wang and Yaqi Fu; photos by Liping Tian; translation by Yanzi Wu; edited by Guojuan Wang, Jacqueline Bánki, and Danny Abbasi
09 Nov 2017
RELATED NEWS
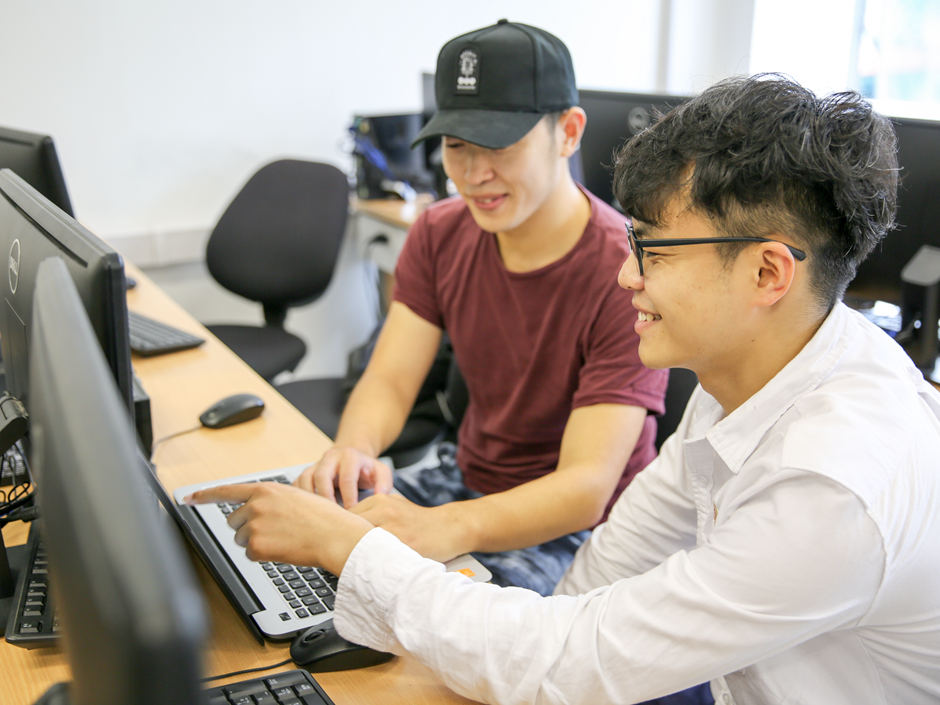
Student team develops classical Chinese translation software
Classical Chinese, also known as Literary Chinese, is the language of classic literature used for more than 2,000 years in China. These days many people have...
Learn more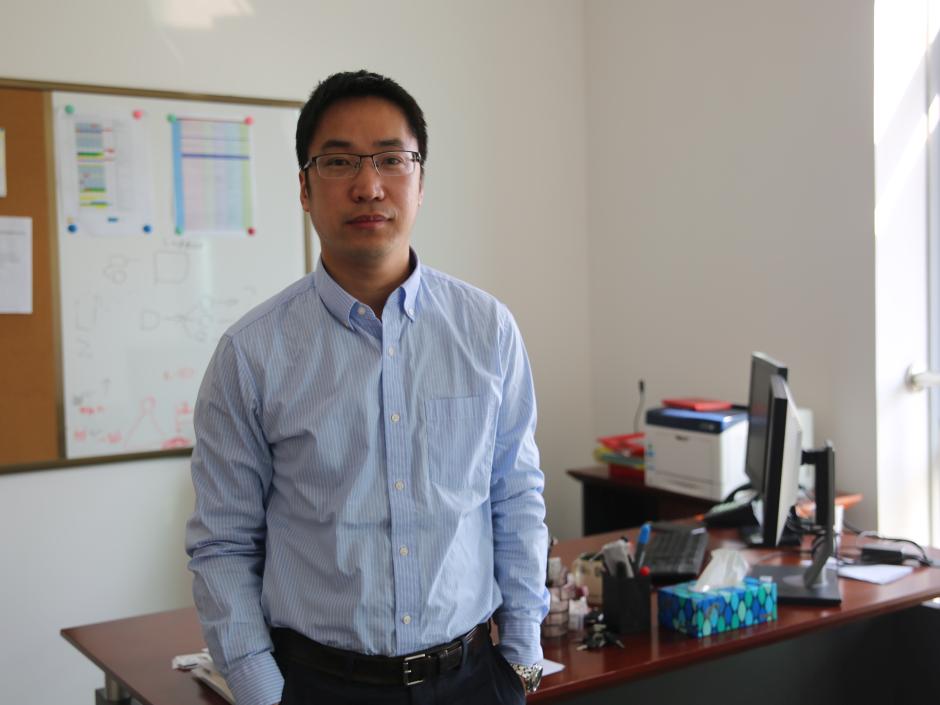
AI research projects at XJTLU: Intelligent scene understanding
It was reported in online magazine The Atlantic that China is becoming the world leader in research into artificial intelligence (AI). Furthermore, global de...
Learn more