25 Jan 2022
We spend more than 90% of our time indoors, so it’s essential that buildings are designed to maximise our comfort, particularly when it comes to maintaining an ambient temperature. Predicting whether a person will be hot or cold in a room is not only important in designing a comfortable space, it can also be useful for reducing energy consumption.
Currently, 55% of a building’s operational energy is spent on heating, ventilation and air-conditioning (HVAC). Machine-learning-based methods are often used to predict individuals’ thermal comfort based on various factors, but the impact of architectural spatial features, such as the position of doors and windows, has not been included in previous modelling.
A fresh perspective
In a recent study published in the Journal of Building Engineering, a team of scientists— including Dr Cheng Zhang and Dr Bing Chen, both from Xi’an Jiaotong-Liverpool University's Design School—sought to address this knowledge gap.
“Our research set out to determine exactly how we can map the thermal comfort of different areas of a room, and how factors such as sunlight exposure, windows, and HVAC positioning affect each area,” explains Dr Zhang. “To this end, we developed an artificial neural network (ANN)-based system to predict personal thermal comfort based on these factors.”
Developing a new model
The team developed the ANN-based model using three main categories of spatial parameters: personal-dependent parameters (age, gender, clothing, body mass index, etc.), environmental parameters (mean indoor temperature, humidity, weather conditions, etc.), and spatial parameters. While the first two have been considered in many personal thermal comfort models before, accounting for spatial parameters is an entirely novel approach to modelling personal thermal comfort.
Dr Chen says: “Most models only cover physical environmental information, with little attention being paid to users’ behaviour within the environment. Our intention was to incorporate human-related factors and behaviour into the existing models.”
Collecting data
Just like for any other machine learning-based approach, the scientists had to train their ANN-based model with real data on all the input parameters and the resulting thermal comfort. The team conducted field experiments in both summer and winter, in five experimental office rooms with different layouts. They also recruited participants who, after staying at a given position in the room for a predetermined time, had to self-assess their thermal comfort. Their forehead temperature was also recorded. The collected data was used to train, validate, and finally test the ANN.
Promising results
The trained ANN model mapped the thermal comfort of a room with exceptional accuracy. The data showed that spatial parameters have a significant impact on the prediction accuracy of the model and determined which spatial factors had the biggest impact on an individual’s thermal comfort.
A path for the future
This research highlights the importance of including architectural spatial features in models to predict thermal comfort and reducing energy consumption, something that has been largely overlooked in previous research efforts.
“Our findings pave the way for future researchers to explore more relationships between indoor spatial layout and individual thermal comfort using AI technology, so as to provide a better environment using less energy,” remarks Dr Zhang. “This may ultimately lead to an acceptable trade-off between thermal comfort and energy consumption achieved through spatial design.”
The research paper, ‘Investigating spatial impact on indoor personal thermal comfort’, was published in the Journal of Building Engineering and can be read in full here.
Edited by Catherine Diamond and Patricia Pieterse
25 Jan 2022
RELATED NEWS
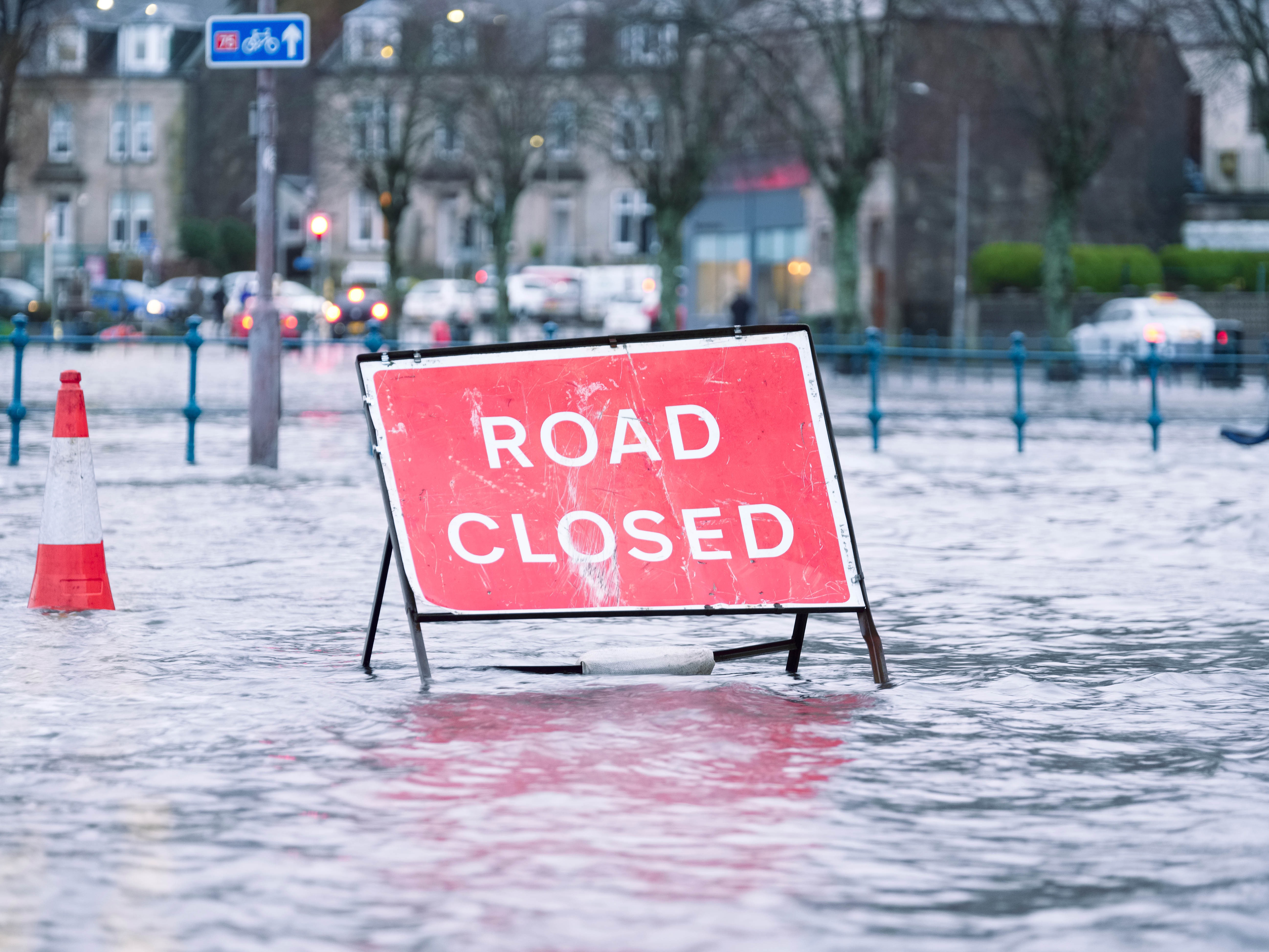
XJTLU study exposes increasing flood risk in the UK
As climate change continues to cause unpredictable and extreme weather events around the world, Xi’an Jiaotong-Liverpool University researchers are calling f...
Learn more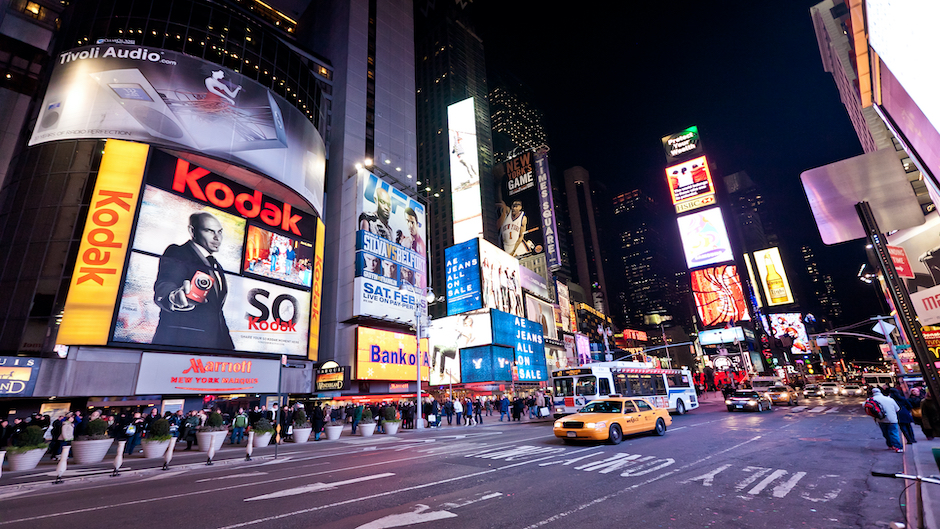
Research: Improved text recognition to aid modern life
Researchers at Xi’an Jiaotong-Liverpool University have proposed a new method to teach a computer to recognise irregular text common in everyday scenarios, s...
Learn more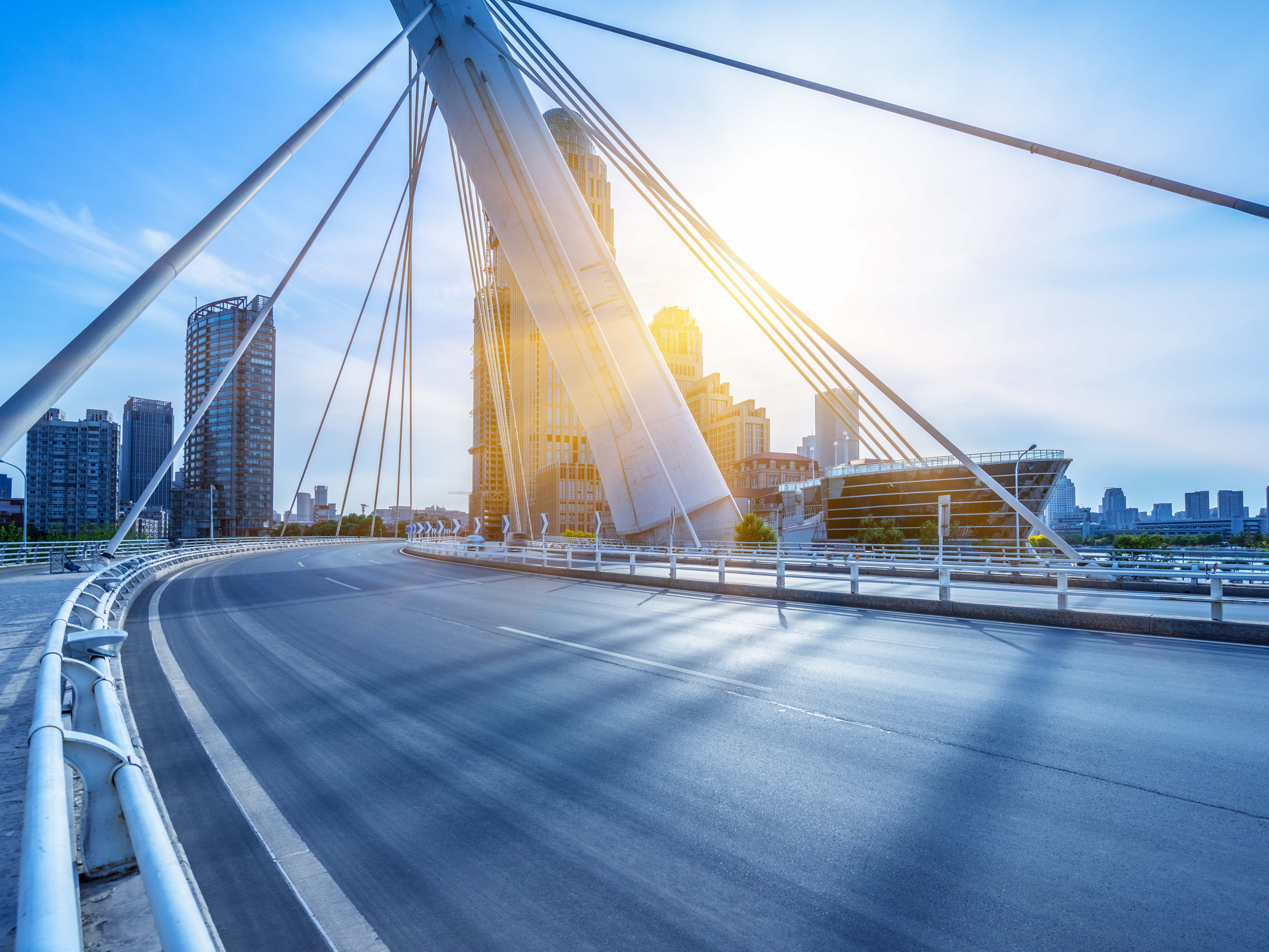
XJTLU researcher unlocks code to calculate structure’s carrying capacity
Have you ever looked up at a skyscraper and marveled at how such an enormous structure can stand without falling over? Or walked across a crowded bridge and ...
Learn more