18 Mar 2022
Rail transit is one of the most energy-efficient modes of transport. However, the railway industry is still responsible for 0.3% of global carbon emissions, and efforts are being made to make it carbon-neutral.
Researchers at Xi’an Jiaotong-Liverpool University have created a new method of calculating metro train timetables to optimise energy consumption. Their model uses artificial intelligence to incorporate real-time passenger numbers into timetabling. It then calculates a train’s recommended speed to avoid delays without a significant rise in energy.
Dr Yida Guo, the first author of the study, says: “Due to the increasing mobility of urban residents, especially during holidays and rush hours, passenger flow has changed greatly. Therefore, we believe a new method is necessary to optimise the train schedule in near real-time to minimise energy consumption.”
Unexpected events
To minimise the amount of energy a train uses, timetables must consider slopes and curves in the track, popular stations, time of day, the distance between stations, and speed limits. They also need to include estimates of the number of passengers getting on and off as this adjusts the time a train spends at each station, the train’s weight, and therefore its energy consumption.
Current timetabling systems that focus on minimising energy consumption are limited. They mainly use ‘offline’ information; this is historical data of passenger numbers and time that a train spends at each station.
However, the timetable cannot be readjusted to account for unexpected disturbances. Consequently, when a train is delayed, the train driver speeds up between stations to catch up, increasing energy consumption. However, this surge may not have been needed as there may be no passengers waiting at subsequent stations.
Dr Guo says: “There will inevitably be some disturbances in train travel – for example, at a certain station, the train stays longer than expected due to too many passengers – which makes it impossible for the train to follow the offline-optimised timetable. Therefore, our research focuses on online optimisation. That is, to re-optimise a timetable for the train in near real-time when it faces a disturbance.”
Overcoming limitations
The few existing models that include real-time information can take up to two minutes to calculate the speed a train should go to catch up with the timetable, without consuming extra energy. During this time, the train may have visited at least one new station and been further delayed by other events, so the solution would no longer be relevant.
To create a model that can optimise energy consumption in near real-time, the XJTLU researchers combine historical ‘offline’ data, including expected passenger numbers and track data (such as slope and curve), with real-time data for passenger flow, including card-swipe data.
They also use AI to identify the number of passengers from images of people waiting on each platform. The model then calculates the train’s expected weight change from this number of passengers. All this data is added to a deep neural network that calculates the necessary speed and timetable for optimal energy consumption.
Testing times
The team of researchers tested their model using hypothetical case studies.
Dr Guo says: “We tested our model using two categories of disturbances with more than 30,000 scenarios. The test results are encouraging, and our model decreases energy consumption by more than 50% compared to previous models when disturbances are encountered. It takes less than one second to calculate this information.”
Although this new model looks promising when used in hypothetical case studies, Dr Guo says there is still a lot of testing that needs to be done before this model can be used in real-life scenarios:
“It is a rigorous process to transform scientific research achievements into engineering applications, especially in the rail transit system, which has strict safety requirements. For example, it is necessary to test how long it takes for the trains to receive the information calculated from the model. Actual test results will then be used to calculate the safety net needed when using this model to ensure passenger safety.”
The research paper, Near real-time timetabling for metro system energy optimization considering passenger flow and random delays, is published in the Journal of Rail Transport Planning & Management, and can be read here.
About the researchers
Dr Yida Guo completed his PhD at XJTLU in 2021, and was supervised by Dr Cheng Zhang.
Dr Cheng Zhang is the corresponding author and is an Associate Professor in XJTLU’s Department of Civil Engineering.
By Catherine Diamond
Edited by Patricia Pieterse
18 Mar 2022
RELATED NEWS
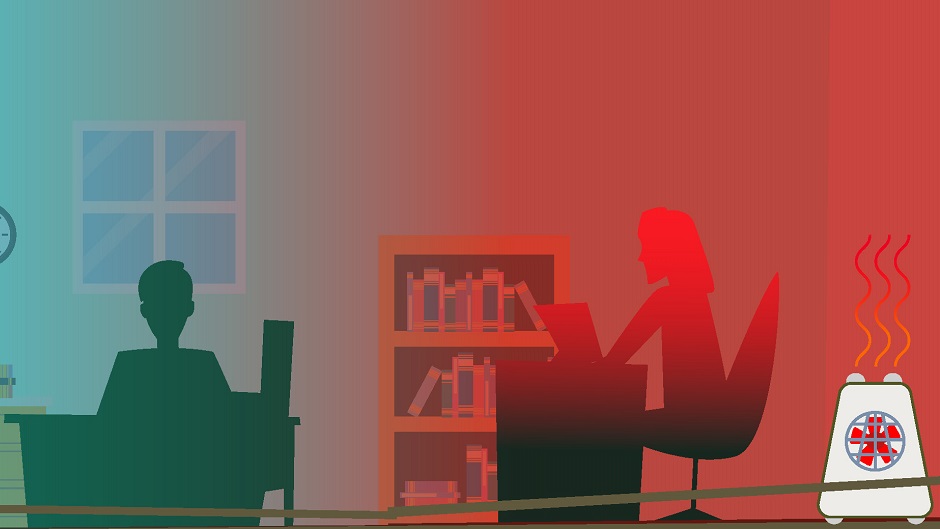
Can AI predict thermal comfort from the layout of a room?
We spend more than 90% of our time indoors, so it’s essential that buildings are designed to maximise our comfort, particularly when it comes to maintaining ...
Learn more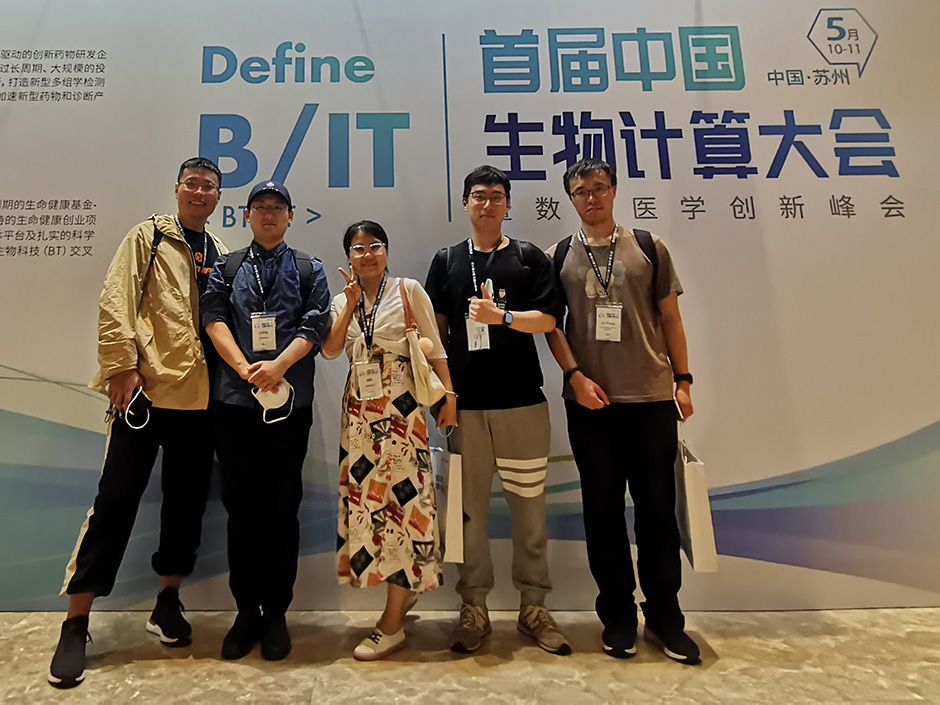
XJTLU student work in RNA modifications in high-impact journal
A Xi’an Jiaotong-Liverpool University undergraduate student has made his academic publishing debut in high-impact journal Nature Communications with a paper ...
Learn more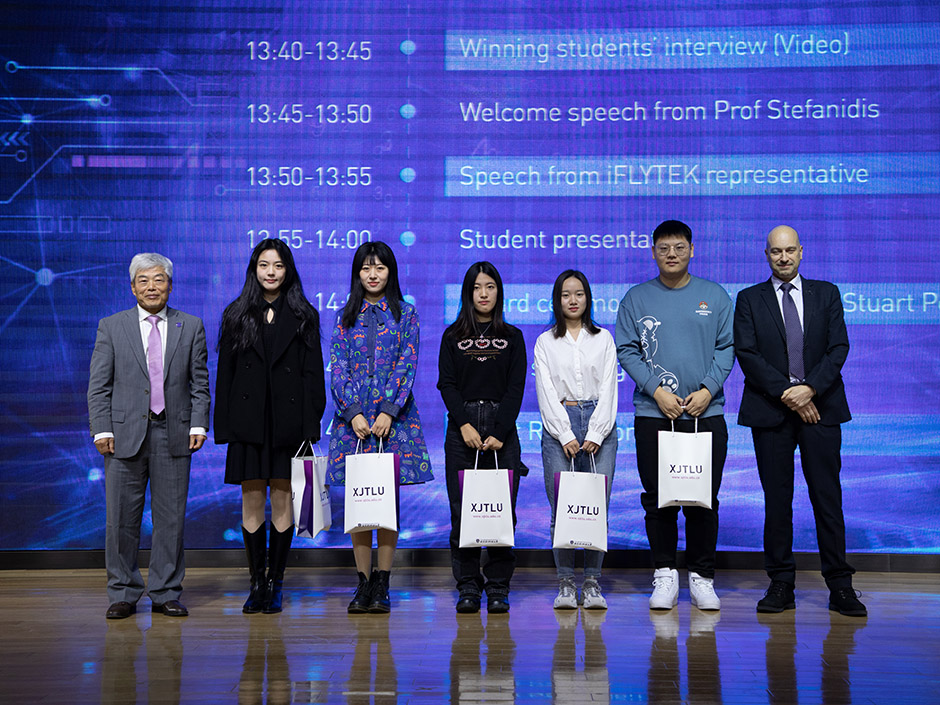
Intelligent car team honoured by XJTLU Entrepreneur College (Taicang)
XJTLU Entrepreneur College (Taicang) recently held a ceremony to honour the student team that won second prize in the iFLYTEK Intelligent Restaura...
Learn more